AI Red Teaming:
Detecting and Combating Political Misinformation
The United States and many other countries face a pivotal election year in 2024. This critical time brings a significant threat to a fair and informed democratic process: the spread of political misinformation. The growing presence of large language models (LLMs) and generative AI (GenAI) has significantly changed how we access and interact with information. Malicious actors can exploit these models to disseminate fake news, manipulate public opinion, and sow discord. From fabricated news stories and deepfakes to social media manipulation, these techniques leverage cognitive biases and emotional triggers to influence public opinion. The consequences are extensive, potentially influencing elections, stoking social unrest, and hindering effective policymaking. Addressing this challenge demands innovative approaches, one of which is AI red teaming.
Understanding AI Red Teaming
Red teaming is a cybersecurity strategy that involves simulating cyberattacks to identify potential vulnerabilities, biases, and undesirable behaviors in the model’s output. In the context of political misinformation, red teaming can be used to proactively assess the potential impact of disinformation campaigns and develop strategies to mitigate their effects. This article focuses specifically on prompt-based red teaming, which tests how an AI model responds to a diverse set of prompts, aiming to elicit undesirable behavior like generating biased or false political content.
How AI Red Teaming Can Combat Political Misinformation
AI Red Teaming offers several advantages in combating political misinformation:
Improved Detection: By simulating real-world disinformation campaigns, AI can help identify emerging trends and tactics used by malicious actors. This allows platforms to develop better detection algorithms and flag suspicious content before it gains traction.
Enhanced Vulnerability Analysis: AI can identify potential weaknesses in existing content moderation systems. By conducting red teaming on these systems with synthetic misinformation, vulnerabilities can be exposed and addressed proactively. This strengthens the overall defense against disinformation campaigns.
Training for Content Moderators: Red teaming generated content can be used to train human content moderators to better identify misinformation. By exposing them to a wider range of tactics and techniques, moderators can improve their ability to discern genuine content from fabricated narratives.
Developing Counter-Narratives: AI can be used to generate counter-narratives that debunk misinformation. These narratives can be tailored to specific disinformation campaigns and targeted towards the same audience – effectively pushing back against the spread of false information.
The Red Teaming Process
Effective red teaming isn’t simply throwing random prompts at an AI. It requires a three-step process:
1. Planning and Threat Modeling: This stage involves a thorough analysis of the model’s purpose, target audience, and potential vulnerabilities.
- Task Taxonomy: Defining the various functions the model is expected to perform (summarization, translation, etc.). Different tasks may have different vulnerabilities to political misinformation.
- Safety Vectors: Defining the types of harm red teaming prompts will attempt to elicit. For political misinformation, this might include prompts designed to generate biased content, fabricate political events, or impersonate political figures.
- Domain Specificity: Developing prompts relevant to the model’s intended use (e.g., a public chatbot vs. an internal election analysis tool).
- Threat Analysis: Considering the context in which the model will be used and the potential actors aiming to manipulate it (e.g., malicious users vs. inadvertent bias).
2. Attack Simulation: Here, the red team uses the developed prompts to test the model’s defenses.
- Task-Specific Attacks: Designing prompts that exploit vulnerabilities in specific tasks. For example, testing a political news summarization model with prompts containing demonstrably biased sources.
- Jailbreaking Strategies: Attempting to bypass the model’s safety measures to generate harmful content. This might involve exploiting the model’s training data or employing “persuasion techniques” that mimic human manipulation. We have a custom taxonomy of techniques that our red teamers use, or we use client-specified techniques.
3. Analysis: Evaluating the results of the attack simulation is crucial. This involves examining the model’s responses to red prompts, identifying successful attacks and areas of resilience.
- Findings Report: A comprehensive report outlining the discovered vulnerabilities, their potential consequences, and recommendations for strengthening the model.
The Future of AI Red Teaming
As AI adoption grows, so will the need for robust red teaming practices. Moreover, government regulations aimed at ensuring responsible AI development will likely emphasize thorough vetting processes, making red teaming an essential tool for those implementing LLMs.
Getting Started with Red Teaming
Red teaming doesn’t have to be an exclusive endeavor. Resources like Innodata’s Model Evaluation Toolkit and datasets can be valuable starting points. These tools can help identify vulnerabilities that can be further targeted with customized model evaluation efforts. For more robust LLMs, Innodata offers expert red teaming services and custom datasets.
Chat with an Innodata expert today about implementing AI red teaming and mitigating risks of manipulation and misinformation.
Bring Intelligence to Your Enterprise Processes with Generative AI
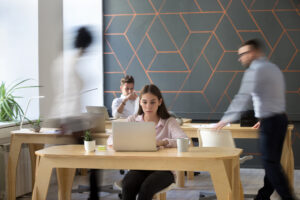
Whether you have existing generative AI models or want to integrate them into your operations, we offer a comprehensive suite of services to unlock their full potential.
follow us
The post AI Red Teaming: Detecting and Combating Political Misinformation appeared first on Innodata Inc..